1. Introduction
The surface electromyogram, sEMG, has been for decades one of the major neural control sources for powered upper limb prostheses [1]. Various sEMG signal processing methods have been used to disclose the user’s intended movement. Conventional myoelectric control schemes employ measures such as the root mean square or mean absolute value of the EMG to quantify the strength of contraction in the underlying muscles [2], controlling a prosthetic device with one or two degree of freedom (DOFs). On the other hand, pattern recognition-based myoelectric control is an advanced signal processing technique that can potentially be used to control multiple DOFs. In this approach, a set of features containing spatial and temporal information related to the acquired signals are extracted to form a pattern that is input to a classifier which determines the user’s intended movement [3].
Although pattern recognition-based control of myoelectric prostheses has deserved great attention in research environments [4,5], it has not been widely used in clinical scenarios. According to scientific literature, myoelectric classification for prosthetic control is not only possible but also highly accurate [4-6]. This conclusion heavily collides with the clinical practice and the existing functional devices [7-9]: amplitude-based myoelectric control (and not pattern classification) has been used in most of commercial devices and only a quarter of patients with upper limb amputations use myoelectric prosthesis [3]. A possible explanation for that could be that researches usually were done under very controlled conditions and some external common factors that exist during daily activities have rarely been considered. In contrast, the changes in pattern during daily activities (caused by electrode displacements, muscular fatigue, variability of muscle contraction effort, limb positions, and many others) can affect the performance and robustness over time of Automatic Control of Myoelectric Prostheses [11]. All of these factors are currently challenges for the clinical use of prosthetic devices. Some newer studies have shown the particular effects caused by electrode displacements [12,13], variability of muscle contraction effort [11], and limb positions [14], however researches on the effects of muscle fatigue have been relatively limited [15]. The focus of this study is to reveal the effects of muscle fatigue on the performance of pattern classification. Muscle fatigue is a major cause of sEMG changes during repetitive contractions performed for long periods of time [16]. It is well known that muscle fatigue changes the recruitment of motor units contributing to muscle contraction, which in turn changes the nature of any sEMG signal measured at that muscle. In muscle physiology, it has been proposed that sustained static isometric contractions may cause an increase in EMG signal amplitude along with a shift of the spectrum toward the low frequencies [17]. For example, on [18], Park and Meek proposed a fatigue compensator preprocessor to reverse the effects of muscle fatigue on the frequency spectrum of an EMG signal. On the other hand, Song et al. [19] found that pattern recognition based systems, such as those that perform the classification using signals from a variety of EMG channels, are especially susceptible to the effects of fatigue.
In this work, we induced muscle fatigue in six non-disabled subjects and studied the effects of EMG patterns modification on LDA classifiers. We found, during muscle fatigue, a decrease in the separability between classes and an increase in the classification error rate. Finally, we compared classification error rate using different training strategies allowing us to claim that an adequate strategy can reduce the effects of muscle fatigue in pattern recognition-based myoelectric control.
2.1. Data acquisition and preprocessing
Surface EMG signals were collected from six healthy, normally-limbed subjects, including three male and three female, with ages ranging from 24 to 36 years. All experiments were approved by UNB Research Ethics Board and all subjects were sufficiently informed about the procedure. Subjects were fitted around the dominant forearm with an elastic band containing six wireless electrodes equally spaced using a Trigno Wireless System (Delsys Inc., USA). The electrodes were placed approximately at one third of the length of the forearm at the area of largest muscle bulk. Data were acquired using a sampling frequency of 2000 Hz with a 16-bit analog-to-digital converter. In order to reduce low frequency motion artifacts, digital data were filtered with a high-pass 3th order Butterworth digital filter with cutoff at 20 Hz and with the Transference Function
Subjects were asked to maintain the contraction of each of eight classes of motion: wrist flexion/extension, wrist pronation/supination, hand close/open, pinch grip and no motion. In order to induce muscle fatigue, sixteen repetitions of each contraction were collected increasing the duration of the contraction: eight repetitions of 3 seconds of duration for each contraction, four repetitions of 10 seconds of duration for each contraction, and finally four repetitions of 30 seconds of duration for each contraction. In all cases 2 seconds of rest were given between each contraction. Fig. 1 is an illustration of how spectral characteristics of EMG signal changed while time of contraction were increased. Note the spectrum shifts toward low frequencies (17.3 Hz in the figure), which is characteristic of muscle fatigue.
Finally, the overall data set included eight repetitions of 3 seconds (no muscle fatigue), four repetitions of 10 seconds (assuming a moderate muscle fatigue) and four repetitions of 30 seconds (assuming muscle fatigue). These data sets were clustered according to the total acquisition time and were named as baseline data, moderate fatigue data and fatigue data respectively.
All the data were collected using a version of the software described in [20]. The program shows the subject the movement to do, control the number of repetitions and duration of each exercise and matches data collected and desired contraction. EMG data were digitally notch filtered at 60 Hz using a 3rd order Butterworth filter in order to reduce power line interference. Data were segmented before feature extraction by applying a 200 ms analysis window [21] with 100 ms of overlap between adjacent segments.
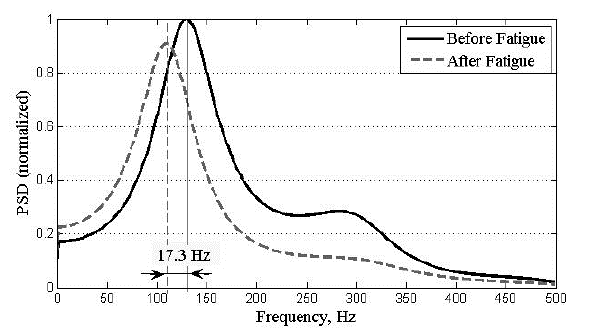
Source: The authors
Figure 1 An illustration of how muscle fatigue was induced by increasing the contraction time.
2.2. Feature extraction and classification
Data were described using three different feature sets: Time-Domain feature set (TD) described in [22], 4th order Autoregressive (AR) features [23-25] and a combination of both (TDAR). Pattern classification was performed using a Linear Discriminant Analysis (LDA) classifier. The TD features used are described by eq. (2)-(5).
Mean Absolute Value (MAV) [26]
Zero-crossing (ZC) [27]
Waveform Length (WL) [22]
Slope Sign Changes (SSC) [22]
In eq. (2)-(5), xi is the sEMG, N in the length in samples of the analysis window.
AR features were obtained by calculating the coefficients,A =[1A(2)…A(K+1) ], of a 4th order [28] forward linear predictor defined by:
in order to minimize the sum of the squares of the errors
More details on TD and AR features extraction can be found in [22] and [23].
2.3. Classification methods
In order to analyze the effect of muscle fatigue on EMG features, we conducted three experiments using different classification schemes. First, data collected in no fatigue condition were used in the training phase and data collected in moderate fatigue and fatigue conditions were used in the testing phase. Second, multiple condition training strategies were used. In this approach data collected in no fatigue, moderate fatigue and fatigue conditions were used for both training and testing. Finally, three LDA classifiers were trained, the first one using data collected in no fatigue condition, the second using data collected in moderate fatigue condition and the last one using data collected in fatigue condition. This approach was named selective classification. Fig. 2 shows schemes of each of these classification methods.
In addition, we propose an adaptive mechanism to improve the performance of LDA classifier when sEMG is affected by muscle fatigue. The proposed method can be summarized as follows: if the resulting classification of each new feature vector is correct the oldest feature vector in the training set is replaced and the classifier is retrained. (See Fig. 3)
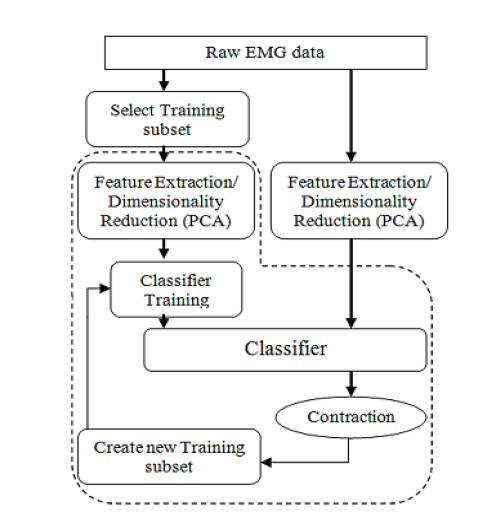
Source: The authors
Figure 3 Flow diagram representing the adaptive LDA approach presented in the current research. Dashed line delimits re-training steps.
In this approach, for each subject the data was divided into two subsets: training data and test data. The training data was used to train a LDA classifier, while the test data was used to evaluate the static LDA classifier and to implement and evaluate the Adaptive Linear Discriminant Analysis (ALDA) classifier.
2.4. Analysis of effects of muscle fatigue
The metrics used to quantify the data characteristics in the feature space were Repeatability Index (RI) and Separability Index (SI), introduced by Bunderson and Kuiken [29].
The RI is a measure of how well a subject is able to reproduce EMG patterns from one trial to the next. The RI is calculated here as one-half the average Mahalanobis distance between the feature vector centroid for a trial (µk,j) and the next trial (µk+1,j), averaged across all the trials and all the active classes as is shown in eq. 8:
Where Sj is the covariance of the data for class j, J is the number of active classes and K is the number of the testing trials. A lower consistency in pattern generation results in a greater RI.
The SI is a measure of interclass distances computed as
In addition to RI and SI, the Total Error Rate (TER) for each of the three classification schemes described above was evaluated, calculated as follows:
In the adaptive approach, the performance of the classifiers was measured by the offline metric classification Accuracy (Acc), False Positive Rate (FPR), Sensitivity (Se) and F1-score (F1). Expressions are given in eq. (11)-(14)
Accuracy:
False Positive Rate:
Sensitivity:
F1-score:
In all cases I is the number of classes considered, I = 8 in this study. In eq. (12) represents the number of false positive when the classification task for each class is considered as a binary problem. The term
is the sum of false positives and true negatives from each class. In eq. (13)
represents the number of true positive for each class i and
is the sum of true positive and false negative for each class. Equation (14) is a simultaneous measurement of sensitivity and precision. Note that for Acc (eq. (11)), Se (eq. (13)) and F1-Score (F1) (eq. (14)) a higher value indicates a major performance, while in FPR (eq. (12)) the best performance corresponds to the lowest value.
3. Results and discussions
Fig. 4 shows the effects of induced muscle fatigue in Repeatability Index (Fig. 4a) and Seperability Index (Fig. 4b). The graphs show the mean value of the index for each feature set and the vertical error bar indicates the inter-subject variability. Note that for visualization purposes indices were normalized. The results showed that both, Repeatability and Separability Indexes decreased when fatigue level increased. The trend is similar in all of the features set considered, but in AR set, the mean of the Separability is the lowest.
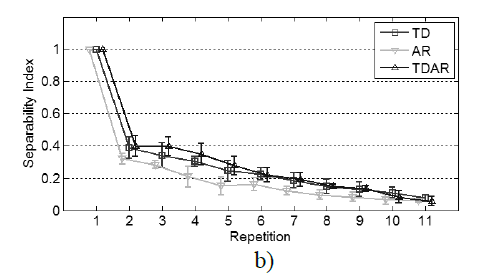
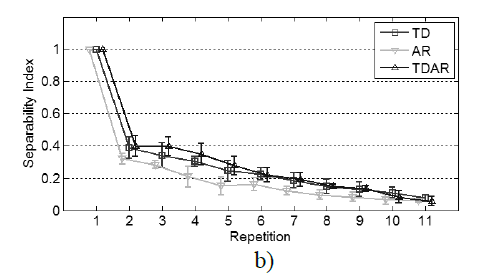
Source: The Authors
Figure 4 Effects of muscle fatigue measure in a) Repeatability Index, b) Separability Index.
The more obvious consequence of this trend in RI an SI, is that the accuracy of the classifier decreases in presence of muscle fatigue. Fig. 5 illustrates the TER when classifier was trained only with patterns from no fatigue condition. In this scheme, the classifier can identify test patterns from non-fatigue data with high accuracy (TER ≈7±2.5%), however it is not able to reliably recognize patterns with moderate (TER ≈21±15%) or high muscle fatigue (TER≈39±15%). The best results were obtained with the TDAR dataset.
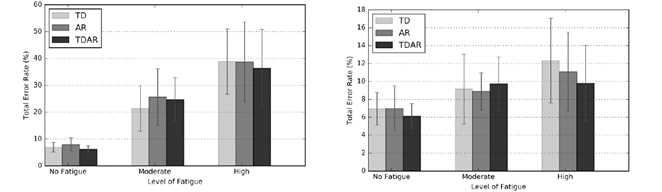
Source: The Authors
Figure 5 Classification Error with no-fatigue data set training, and test was performed with fatigue and no fatigue condition.
The results of Multiple Condition Training (Fig. 6) showed that TER in moderate and high levels of fatigue decreased to 9.3 ± 1.4% and 11.9 ± 0.95% respectively. This is around 25% better compared to the previous scheme. In contrast, in no fatigue data TER increased to 8.67 ± 2.5%. This trend is similar in all of the features set analyzed. These results suggest the need of a new scheme based on selective classification.
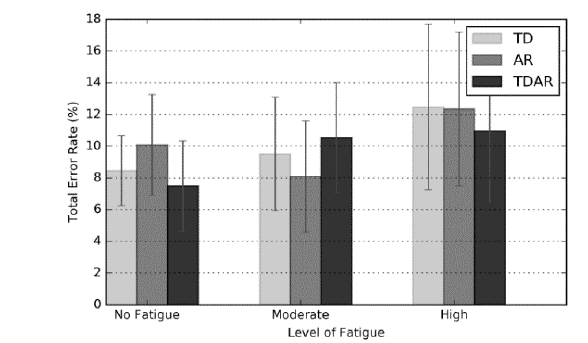
Source: The Authors
Figure 6 Classification Error when classifier was trained using Multiple Training Scheme and test was performed using data in no fatigue, moderate and high fatigue condition.
Results for the Selective Classification scheme are showed in Fig. 7. A simple analysis of the figure suggests that the classifier achieved stable results for all data sets with this approach. However, with no fatigue data set the classifier did not increase the accuracy. This is because the data used to train the classifier were the same used in the first training scheme. Using the dataset with moderate level of fatigue the classifier showed a TER of 9.26%, while the data with high level of fatigue showed a TER of 11.06%. It represents an increase in accuracy compared with the traditional approach.
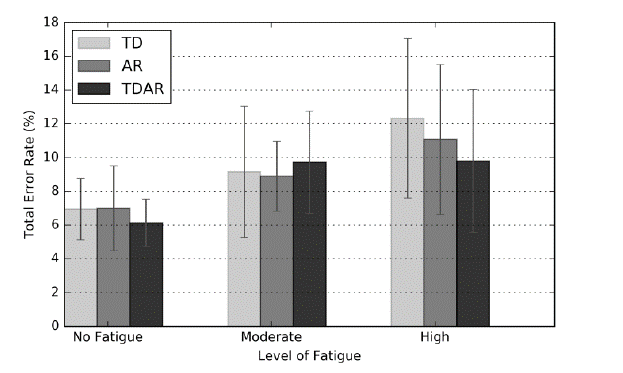
Source: The Authors
Figure 7 Classification Error when classifiers were trained using Selective Classification Scheme and test was performed using data in no fatigue, moderate and high fatigue condition.
Classification results were validated using Accuracy (Acc), False Positive Rate (FPR), Sensitivity (Se) and F1-score (F1). Validation parameters were calculated epoch by epoch in both scenarios: using Adaptive LDA and using non-adaptive LDA. Fig. 8 represents the mean and standard deviation of the parameters for the six subjects. Parameters were obtained from each of the class and combined. The solid lines represent classification results of the adaptive LDA classifier proposed in the current work, whereas the dashed lines represent classification results of the conventional LDA classifier. As shown in Fig. 8, when muscle fatigue increases, the accuracy and sensitivity of the conventional LDA classifier decreases from more than 90 % to less than 58 % in normally-limbed subjects. Fig. 8 (b) shows that False Positive Rate increases from around 9% to 36.2 %. The F1-score (Fig. 8 c) decreases from 0.9 to 0.6. On the other hand, the parameters of the adaptive LDA show stable and higher performance.
4. Conclusions
In this study we investigated the effects of muscle fatigue on the performance of automatic pattern recognition of eight movements of the arm by using the repeatability and separability indices and the classification rate. It was shown how muscle fatigue affects the feature spaces and also the classification rate. Total Error Rate computation for the schemes addressed demonstrated how the use of a multiple condition training scheme can reduce the classification error in presence of muscle fatigue, but it also degrades the performance of the classifier when data are not affected by fatigue. On the other hand, selective classification improves the performance of classifier in presence of muscle fatigue without affecting the performance in absence of fatigue. Nevertheless, this solution presents two major problems: the first one is the need of training three different classifiers which increases the computational load; the second, and more important, is the need to previously assess the fatigue condition, requiring an additional classifier to determine the fatigue level in a dual-stage classification scheme. In the case of adaptive vs non-adaptive LDA, results show that when muscle fatigue increases, the recognition accuracy and sensitivity of the non-adaptive LDA classifier decreases from more than 90% to less than 58% in normally-limbed subjects, in the same situation False Positive Rate increases from around 9% to 36.2% and the F1-score decreases from 0.9 to 0.6. These parameters showed a more stable behavior and higher performance when adaptive LDA was evaluated. Future work should respond to the question: when it is necessary to adapt the classifier?