1 Introduction
The measurement of soil moisture and soil water tension are common practices in the research activities and irrigation management of crops. The standard method for measuring soil moisture involves weighing a soil sample before and after drying it in an oven at 105 °C. Although accurate, the gravimetric method is relatively time-consuming and sometimes requires a lot of manpower to execute, especially when there is a need for measurements at different locations and soil depths. Commercially, there are several sensors that measure soil moisture quickly and accurately; they include some electronics, such as the Diviner 2000® profiling probes (Sentek, Stepney, Australia), and PR2 (Decagon Devices, Pullman, Wash.), and other sensors, such as ML3 (Decagon Devices, Pullman, Wash.). The quality of the measurement data obtained with this equipment has been demonstrated in studies [6,7]. However, the high cost of acquisition often limits its use.
Soil water tension is usually determined using tensiometers, which can be puncture tensiometers, with analog vacuometers, or with mercury columns. In tensiometers with coupled vacuometers, measurements are obtained directly from the equipment. In the puncture tensiometer, measurements are performed using digital or analog tensiometers. Regardless of the type of tensiometer used, the large-scale use of this equipment has a relatively high cost, especially when using the vacuometer.
Recently, with the advancement of electronics, several sensors have emerged in e-commerce, which can be used with an Arduino® board. These instruments have gained popularity because of the low cost of acquisition and maintenance, which allow them to be used widely. For measuring soil moisture, there are resistive and capacitive sensors that are becoming increasingly popular in agricultural applications. These sensors have been specifically tested in irrigation automation [5] and soil moisture measurement in the field [4]. For example, a YL-69 resistive soil moisture sensor was evaluated and compared with a commercial ECH2O sensor [4]. According to previous research [4], the YL-69 sensor has excellent performance if it is calibrated for the soil under study.
Studies are still incipient for low-cost capacitive soil moisture sensors (commercially named “Capacitive Soil Moisture Sensor v1.2”). During experiments, the assessments are usually made by subjecting the sensor to a range of water content to obtain a soil-specific calibration equation and the physical condition of the soil. A study was conducted to evaluate the use of these sensors for automated soil moisture monitoring in organic-rich gardening soil [13]. The root-mean-square error (RMSE) of the soil water content obtained with the capacitive sensor function was 0.07 cm3 cm−3 for samples in the dry to saturated range. However, the performance of capacitive sensors in organic and mineral soils can be considerably different owing to differences in electrical conductivity and porosity [14].
The MPX5100DP pressure transducer has been used as an alternative for determining soil water tension measurements. This sensor provides a linear voltage output for the differential pressure range 0-100 kPa [3] and has been used successfully in the agricultural sector [1,8]. By calibrating the pressure transducer with the capacitive soil moisture sensor, it is possible to automatically obtain the initial part of the soil water retention curve, which is extremely important information for the purposes of irrigation management and scientific research. This calibration would also allow the use of several sensors in conjunction with a relatively low cost, in addition to enabling the acquisition of data with a higher temporal frequency.
Thus, the objective of this study is to calibrate and validate the low-cost capacitive sensor "v1.2" and pressure transducer MPX5100DP for measuring soil moisture and determining the initial water retention curve in Red Oxisol, which has a clay texture.
2 Materials and methods
The experiment was conducted at the Federal University of Rondonópolis, Mato Grosso State, Brazil. The soil used was Red Oxisol with a clay texture (41 % clay, 40 % sand, and 19 % silt), collected in the experimental area of the campus and sieved in a 3 mm mesh.
The sensor used for measuring soil moisture was the “Capacitive Soil Moisture Sensor v1.2;” the pressure transducer MPX5100DP coupled to a tensiometer was used for monitoring soil water tension (Fig. 1). These sensors were connected to an Arduino Uno board, along with a micro-SD card module for storing data. The connection schemes of the sensors are shown in Fig. 2.
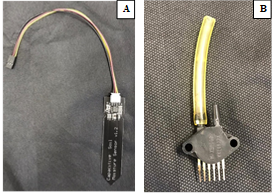
Source: The authors.
Figure 1 Capacitive soil moisture sensor v1.2 (A) and pressure transducer MPX5100DP (B) used for monitoring soil moisture and soil water tension.
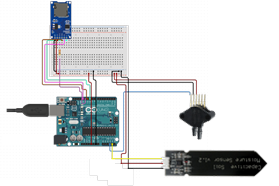
Source: The authors.
Figure 2 Wiring diagram for capacitive sensor and MPX5100DP pressure transducer, with data storage on a memory card in the SD module.
To calibrate the capacitive sensor, PVC (polyvinyl chloride) cylinders that were 150 mm in diameter and 100 mm in height were used, with three repetitions. The soil inserted in each ring was the amount needed to reach a soil bulk density of 1.20 Mg m-3, a value representative of the local soil.
After assembly, the PVC cylinders were placed in a pot with water for soil saturation, and later, capacitive sensors were inserted in the center of each cylinder. Daily, the variation in the mass of the cylinder + soil + sensor set was measured using a digital scale with a resolution of 0.1 g; the output signal of the capacitive sensor was recorded. Thus, using these measurements, the data pairs of the gravimetric soil moisture (θm) and capacitive sensor signal were obtained.
Subsequently, three calibration equations were adjusted for the data set. These equations were chosen because they represent a curvilinear relationship between the dependent and independent variables, similar to what occurs during soil drying. The equations adopted were as follows:
where:
θm = soil moisture (kg kg-1)
a, b, and c = model adjustment parameters.
x = output signal of the capacitive sensor.
In another stage, three PVC cylinders with diameters and heights of 200 mm and 100 mm, respectively, were assembled using the previously described assembly procedure. These cylinders were used to measure the soil moisture with the capacitive sensor and validate the calibration equations obtained previously (Eqs. 1, 2, and 3).
In the same PVC cylinder (200 mm), tensiometers were inserted to measure the soil water tension using the MPX5100DP pressure transducer (Fig. 3A). To use the MPX5100DP pressure transducer, an adaptation was made in the tensiometers to allow the measurement of the soil water tension using a digital tensimeter (SondaTerra®, range 0-96 kPa, 2 % precision) and a pressure transducer simultaneously. The adaptation consisted of connecting a 5 mm diameter silicone hose to the tensiometer PVC tube (Fig. 3B). The connection between the hose and pipe was carefully sealed to ensure that there were no air inlets.
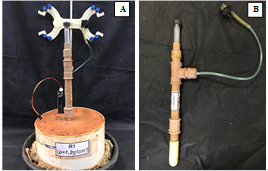
Source: The authors.
Figure 3 Adaptation of the tensiometer to connect the pressure transducer (A); Assembly of the experimental unit containing the tensiometer connected to the pressure transducer and the capacitive sensor (B).
These PVC cylinders were weighed daily with a digital scale (capacity 10 kg, precision 1 g) to determine the gravimetric water content, and concomitantly, data from the capacitive sensor and pressure transducer were obtained. Using the soil moisture and soil water tension data, two water retention curves were constructed: one using the measured data with the capacitive sensor (using the calibration equation with the best performance after the validation step) and the other using the data obtained by weighing.
For the water retention data, three mathematical models, which express the relationship between soil moisture (θ - kg kg-1) and soil water tension (Ψ − kPa), were adjusted. The models were implemented in the SWRC software, which is a software for adjusting soil water retention curves [10].
Exponential model:
Power model:
where Ψ is pressure head units (kPa); β and α are shape parameters.
Van Genuchten (1980) model:
where θs and θr are the saturated and residual water contents (kg kg−1), respectively; α, n, and m are shape parameters, and m is assumed to be m = 1 - 1/n.
The evaluation of soil moisture estimation models was based on the statistical indices, coefficient of determination (R2), the agreement index of Willmott (d), mean absolute percentage error (MAPE) and root mean square error (RMSE).
where Oi, Pi, N, and Ō represent the measured value, predicted value, total number of observations, and the mean of the measured values, respectively.
3 Results and discussion
The relationship between gravimetric soil moisture based on weighing and capacitive sensor signal is shown in Fig. 4. The measured soil moisture and capacitive sensor signal were in the range 0.40-0.06 kg kg−1 and 336-516, respectively. Thus, a wide data range, which is required for sensor calibration, was achieved. The three calibration equations fitted to the data obtained a determination coefficient (R2) above 0.93, MAPE of less than 12%, and RSME of 0.029 (Table 1). However, the function y=1⁄(a+bx) showed a better fit than the others, with a MAPE of 8 %, RMSE of 0.019, and R2 of 0.96.
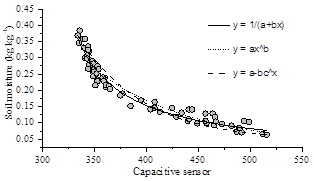
Source: The authors.
Figure 4 Relationship between gravimetric soil moisture (θm - kg kg-1) and the output signal of the capacitive sensor v1.2.
Table 1 Parameters and statistical analysis of the fitted models for determining soil moisture as a function of the capacitive sensor signal.

SE: standard error.
Source: The authors.
The second evaluation stage for the capacitive sensors consisted of validating the calibration equations. There is a linear relationship between the soil moisture measured by weighing, considered as the standard method, and that determined with the capacitive sensor, using the three calibration equations fitted previously (Fig. 5). Although the verified maximum error was 12 % and 0.039 (MAPE and RMSE, Table 2), it is verified that generally, there is a slight tendency of the capacitive sensor to underestimate the soil moisture in comparison with the standard method, especially at higher values.
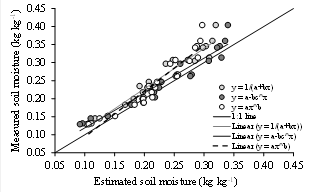
Source: The authors.
Figure 5 Relationship between soil moisture (θm - kg kg-1) measured by gravimetric method and determined with the capacitive sensor.
Table 2 Performance evaluation of the fitted models for the determination of soil moisture as a function of the capacitive sensor signal.

Source: The authors.
This greater dispersion with increasing soil moisture has also been verified by other authors with other capacitive sensors [15]. In a study evaluating two commercial sensors, one of the frequency domain reflectometry (FDR) type and the other of the high-frequency soil impedance (HFSI) type, it was found that both methods showed good correlation compared to the standard method (gravimetric) [7]. However, the HFSI probe overestimated the soil moisture values when compared to the gravimetric method, whereas the FDR underestimated the values. The errors verified by the authors were between 0.06 and 0.08 (RSME). Therefore, they were greater than the errors found in this study. Another study found that the sensitivity of the capacitive sensors EC-5 and EC-20 decreased with increasing soil moisture or permissiveness of the medium [16]. Thus, in general, capacitance probes operate at relatively low frequencies, which makes them less expensive but more sensitive to the confounding effects of salinity, temperature, and soil textural variations [14].
Another factor that may have contributed to the small differences between the soil moisture obtained with the capacitive sensor and that obtained with standard method can be attributed to the area of influence of the sensor. Whereas the moisture measurement by weighing considers the entire volume of soil, the measurement with the sensor depends on its actuation diameter, which may have caused the small differences, especially for the higher moisture values. The diameters of influence for several commercial sensors (TDR100, Theta probe, Hydra probe, 5TE, SM300, and CS616) were evaluated and it was found that the minimum soil sampling diameter of these sensors ranged from 3 cm to approximately 12 cm [14].For capacitive sensor v1.2, there are no published results on the volume of influence for the sensor [17].
After the validation step, considering the statistical indices (Table 2), the calibration equation that obtained the best performance was y=a-bcx with values of 9 % (MAPE), 0.025 (RMSE), 0.97 (d), and 0.93 (R2).
The relationship of the soil water tension measured by the pressure transducer and digital tensimeter showed a coefficient of determination (R2) of 0.98 (Fig. 6). The capacitive soil moisture sensor and pressure transducer were used for the concomitant measurement of soil moisture (calculated using the calibration equation y= a - bcx) and soil water tension, respectively. Subsequently, the initial part of the water retention curve was obtained.
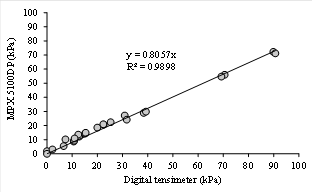
Source: The authors.
Figure 6 Relationship between soil water tension measured with MPX5100DP pressure transducer and with the digital tensimeter.
The water retention data obtained with the capacitive sensor and by weighing, as well as the models fitted to the data, are shown in Fig. 7. It can be seen that the two methods of obtaining the retention curve obtained values that are almost similar. In general, the errors of the two retention curves were 9.2 % (MPAE) and 0.025 (RMSE).
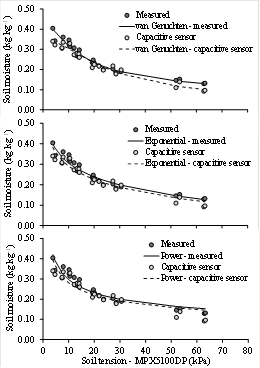
Source: The authors.
Figure 7 Soil water retention curve obtained with the capacitive sensor and by gravimetric method, fitted to different models.
Regarding the retention models, Tables 3 and 4 show the fitted parameters and the statistical analysis of the fitting, respectively. All models obtained a good fit, which was confirmed by the statistical parameters (Table 4). The model with the largest error was the power type, both for the data obtained by weighing and for the data obtained with the capacitive sensor. In addition, with the fitted models, the soil moisture was simulated at two pressures (10 kPa and 33 kPa), commonly defined as field capacity. The differences between the values calculated for each method (Table 5) ranged from 0.01 to 0.02 kg kg-1 (absolute difference), and from 4 to 7 % (relative difference).
Table 3 Model parameters fitted in soil water retention curve obtained with gravimetric method and with capacitive sensor.
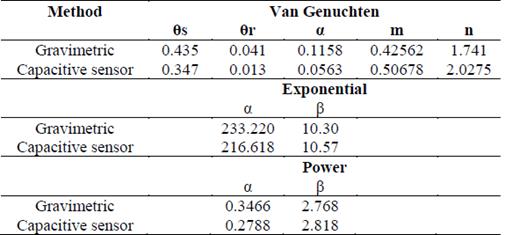
Source: The authors.
Table 4 Statistical parameters of soil water retention curve models fitted in gravimetric and capacitive sensor data.
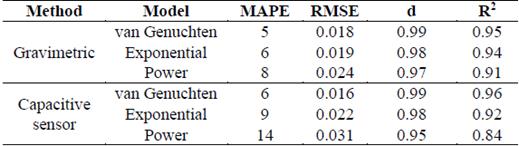
Source: The authors.
Table 5 Soil moisture calculated with different models in two pressures defined as field capacity.
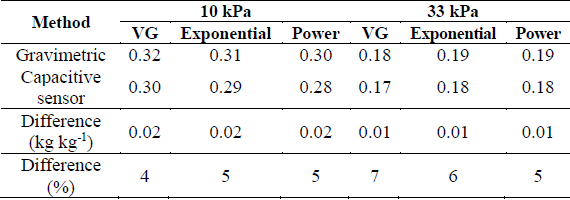
VG: Van Genuchten model.
Source: The authors.
The capacitive sensor under study was used to evaluate an organic soil (24.8 % organic matter; ρd = 0.6 g cm-3) with dry to saturated levels of soil water content; the general measurement error (RMSE) was 0.09 [13]. In addition, the authors evaluated the sensor only in a soil moisture range close to the field capacity (gravimetric water content of 60 %-80%), with an error of 0.05 (RMSE).
The determination of the soil water retention curve (SWCR) for soils subjected to lower pressures is usually carried out in the laboratory by applying suction using a hanging water column or applying pressure above the soil sample using pressure plates. These methods require the collection of several samples and punctual measurements, and they take several days to execute. Thus, the use of a capacitive sensor with a pressure transducer is a promising low-cost alternative that makes it possible to obtain continuous data. In Fig. 8, an example of automatic data collection of soil moisture and soil water tension is presented using the sensors evaluated in this study. This high frequency of data collection has various advantages, such as the construction of a better soil water retention curve owing to the improved measurement ranges for estimating model fitting parameters [18].
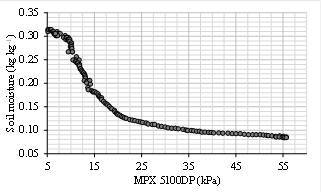
Source: The authors.
Figure 8 Example of continuous measurements of soil moisture and soil water tension with the associated capacitive sensor v1.2 and the pressure transducer MPX5100DP.
In field conditions, the relationship between moisture and soil water tension is measured to determine various physical properties of soil, such as field capacity and hydraulic conductivity, using the instantaneous profile method [11]. In this case, the automation of measurements is also an advantage because it increases the amount of data collected, with the possibility of storage, which makes the method less laborious.
Recently, a study was conducted to determine the water retention curve in the field using soil moisture measurement sensors and MPS-6 (soil tension measurement) sensors [12]. The error (RMSE) compared with the traditional laboratory method ranged from 0.11% to 23 %. The authors verified the tendency of the sensors to underestimate soil moisture in comparison with laboratory measurements. This difference was attributed to possible air trapping in the soil.
The relationship between soil matric potential and soil moisture is soil-specific [9]. The two retention curves obtained by the different methods (weighing and capacitive sensors) were similar (Fig. 7). The greatest differences were in the van Genuchten model, especially at the highest pressures. Despite this, the low-cost sensors used in this study have the potential to obtain data on moisture and soil water tension, and consequently, the construction of the initial part of the characteristic curve. The automation of these measurements is a trend that is growing and is the objective of many studies. The HYPROP combined with the WP4 sensor was used to determine the retention curve in three soils with different textures, compared with the traditional method (hanging water + tempe cell + pressure plate) [9]. For silty clay textured soil, the methods did not provide a good match. However, for the other two soils (silty loam and sandy loam texture), the absolute error was less than 2% for the two methods.
4 Conclusions
The low-cost “Soil Moisture Capacitive Sensor v1.2” and MPX 5100DP sensors were evaluated for soil moisture and soil water tension measurements. The capacitive sensor was calibrated and validated for Red Oxisol, with a clay texture and a bulk density of 1.2 Mg m-3. Among the fitted equations, θm=a-bcx exhibited the best performance. However, there is still a slight tendency to underestimate the soil moisture for higher values, the causes of which still need to be investigated. Calibrated with the MPX5100DP pressure transducer, it was possible to obtain the initial part of the water retention curve; the difference in results for the reference method (based on weighing) and the sensors was 0.025 (RMSE). The low cost of the sensors, the possibility of automating measurements, and the performance of measurements make these sensors a promising alternative to high-cost sensors.